Foxes vs Hedgehogs: Classifying Countries as Generalists or Specialists Based on Entropy Scores
Introduction
This analysis draws its inspiration from Charles J. Gomez's previous research, available at https://doi.org/10.1016/j.respol.2024.105040
The analysis uses Shannon Entropy as a measure of how “generalist” or “specialist” a particular country is with respect to topic distributions. High entropy values indicate a more even (generalist) distribution across multiple topics, whereas low entropy values indicate a more uneven (specialist) distribution, focusing on fewer topics.
We calculate entropy in two primary ways:
Using Topic Prevalence:
Here, we measure the proportion of citations associated with each topic.
Non-significant topics (with P-values greater than 0.1) can be either zeroed out or entirely removed, affecting the resulting distribution.
Using (Beta Coefficient Estimate + Intercept of STM’s regression model for the country covariate):
Here, each topic is associated with a regression estimate (and an intercept).
Non-significant topics are set to 0 in this approach, thereby not contributing to the entropy calculation.
These two approaches yield different plots and interpretations, described below.
Entropy Calculation
Defining calculate_entropy(vec)
:
Probabilities
prob_vec <- vec / sum(vec)
Each entry in
vec
is divided by the sum of the vector, giving a probability distribution.Raw Entropy
entropy = -∑(p_i * log2(p_i))
where each
p_i
is the probability of the i-th topic.Uniform Entropy
If there are N topics, the uniform probability is 1/N. Therefore:uniform_entropy = -∑[ (1/N) * log2(1/N) ] (from i = 1 to N)
Normalized Entropy
normalized_entropy = 1 - (entropy / uniform_entropy)
A value near 0 indicates high specialization (low entropy).
A value near 1 indicates high generalization (high entropy).
Below is the R function used to calculate entropy measures:
calculate_entropy <- function(vec) {
prob_vec <- vec / sum(vec)
# Calculate the entropy of the vector
entropy <- -sum(prob_vec * log2(prob_vec), na.rm = TRUE)
N <- length(vec)
uniform_entropy <- -sum(rep(1/N, N) * log2(rep(1/N, N)))
# Normalize the Entropy Score
normalized_entropy <- 1 - (entropy / uniform_entropy)
return(normalized_entropy)
}
Plots Description
Plot 1
X-Axis: Topic Prevalence
All topics’ prevalence values are included, even those with non-significant estimates (which would be 0 if you tried to combine estimate + intercept).
Y-Axis: Shannon Entropy Scores (calculated from topic prevalence)
Entropy computed across all topics for a given unit (e.g., country and time period).
Data Points: Covariates
Each data point represents one (Country, Time Period) combination, grouped by the covariate in question.
We have 5 time intervals, hence 5 data points per country.
Plot 2
X-Axis: (Estimate + Intercept)
Non-significant estimates are explicitly zeroed out.
Y-Axis: Shannon Entropy Scores (calculated using Estimate + Intercept, with non-significant estimates = 0).
Data Points: Covariates
Again, each data point is grouped by time period and covariate, giving 5 data points per country.
Interpretation:
Here, you are visualizing how different weighting (via regression estimates) might reflect topic diversity.
Topics that are non-significant do not contribute, essentially shrinking the distribution’s breadth.
Plot 3
Purpose: Compare measures of generalist–specialist across countries, using two different methods of weighting topic distributions:
Topic Prevalence (% deflated citation proportion).
(Estimate + Intercept) (with non-significant estimates = 0).
Y-Axis: Generalist–Specialist score (i.e., Shannon Entropy).
X-Axis: Countries
Likely each country’s position on the generalist–specialist continuum is shown.
Handling of Non-Significant Topics:
For topic prevalence: you are removing non-significant topics entirely. (You found that zeroing them out flattens the distribution, so you excluded them to avoid the flat-line problem.)
For estimate + intercept: non-significant topics remain, but are set to 0 in the estimate.
Interpretation:
By comparing these two approaches, you can see whether countries that appear “generalist” under topic prevalence remain “generalist” under the regression-based weighting, and vice versa.
A flat line under the topic prevalence approach with zeroed-out non-significant topics indicates the distribution might be dominated by just a few large topics or that the zeroing out artificially collapses topic diversity.
Interpreting High vs. Low Entropy
High Entropy → Generalist
A high normalized entropy score (closer to 1) means the topics (based on either prevalence or estimate + intercept) are more evenly spread out, indicating a “generalist” pattern.Low Entropy → Specialist
A low normalized entropy score (closer to 0) means the distribution of topics is skewed toward one or a few dominant topics, indicating a “specialist” pattern.
NOTE* - Deciding whether to remove or zero-out non-significant estimates depends on the research question:
If the presence/absence of a topic is itself meaningful, you might remove the topic altogether if it is not supported (i.e., non-significant). In that case, you recalculate probabilities only among the “significant” topics.
If you believe every topic can exist at some level, but the regression result for that topic is effectively zero, you might want to keep the topic as zero to show it does not contribute to the distribution.
However, setting many topics to zero can cause the denominator (sum of probabilities) to rely heavily on a few topics and potentially flatten the entropy measure. If the flat-line pattern does not make theoretical sense, it may be preferable to remove non-significant topics or think carefully about thresholds for significance.
Workflow
Topic Prevalence Workflow
Drop non-significant topics from your dataset.
Calculate
calculate_entropy()
on the remaining topics.Plot the resulting normalized entropy scores vs. topic prevalence or time.
(Estimate + Intercept) Workflow
Set non-significant estimates to 0.
Sum over all topics (including zeros) to get a distribution.
Calculate entropy from this distribution.
Plot the normalized entropy scores vs. (Estimate + Intercept).
Compare & Contrast
Use Plot 3 to see how the two methods (Prevalence-based vs. Estimate-based) may yield similar or different “generalist”/“specialist” rankings for countries.
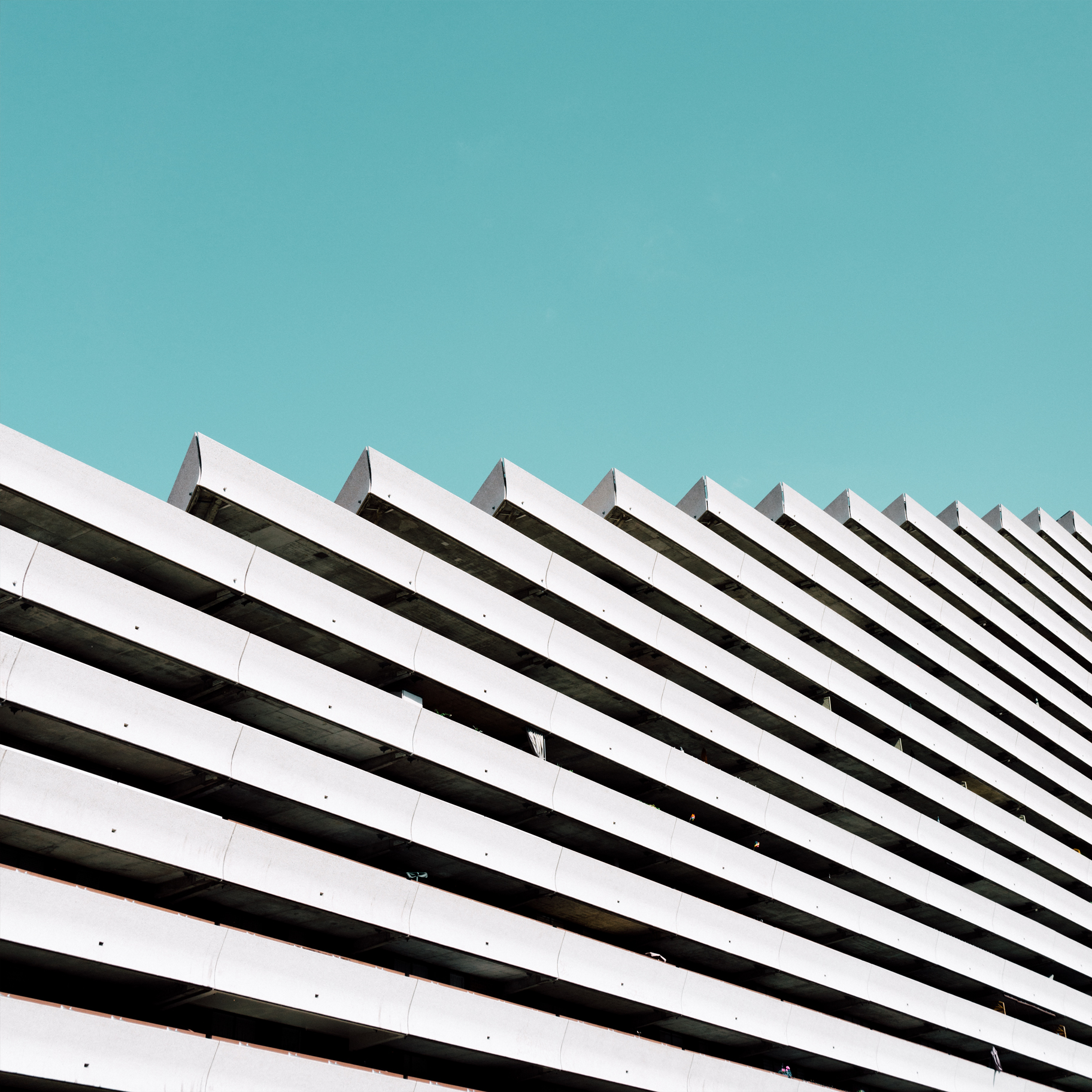